Abstrak
Penelitian biasanya difokuskan pada bagaimana jenis usaha tertentu dikaitkan dengan lokasi kejahatan di blok jalan. Namun, penelitian dalam genre ini sering kali tidak memperhitungkan konteks bisnis umum dari blok tempat bisnis berada. Penelitian ini menggunakan sampel blok yang besar di California Selatan untuk menguji apakah konteks bisnis penting. Kami menilai apakah ada hubungan nonlinier antara total bisnis di blok dan kejahatan, apakah ada perbedaan berdasarkan kategori bisnis yang luas—bisnis yang berhadapan dengan konsumen, bisnis kerah biru, dan bisnis kerah putih—dan apakah pencampuran bisnis di blok memengaruhi kejahatan. Penelitian ini menemukan bukti kuat bahwa blok dengan lebih banyak pencampuran bisnis memiliki tingkat kejahatan yang lebih tinggi. Peningkatan 1 standar deviasi dalam pencampuran bisnis dikaitkan dengan 35%–95% lebih banyak kejahatan. Hubungan antara pencampuran bisnis dan kejahatan dimoderasi oleh ukuran populasi di blok tersebut. Bukti juga menunjukkan perbedaan dalam hubungan dengan kejahatan antara bisnis yang berhadapan dengan konsumen dan bisnis kerah putih atau biru. Hanya bukti sederhana yang menunjukkan bahwa jenis bisnis tertentu terkait dengan tingkat kejahatan setelah memperhitungkan konteks bisnis umum ini.
1. PENDAHULUAN
Bisnis memainkan peran integral dalam masyarakat modern karena mereka tidak hanya menyediakan barang dan jasa bagi penduduk tetapi juga lapangan kerja. Meskipun ada manfaat ini, penelitian secara konsisten menunjukkan bahwa kehadiran mereka berdampak pada tempat terjadinya kejahatan, setidaknya sampai batas tertentu. Teori kriminologi sering menjelaskan hubungan ini lebih berkaitan dengan peluang kejahatan daripada hal-hal yang tidak diinginkan dari bisnis. Mengingat bahwa bisnis yang berbeda memiliki fungsi yang berbeda—dan bahkan berbeda dalam tingkat di mana mereka secara langsung melayani masyarakat—oleh karena itu, bisnis tersebut seharusnya berdampak berbeda terhadap kejahatan, dan penelitian sering kali berfokus pada pertanyaan ini (Gorman et al., 2001 ; Roncek & Maier, 1991 ). Meskipun demikian, tantangan utama penelitian adalah bagaimana memperhitungkan ekologi bisnis yang lebih luas di suatu lingkungan saat mengeksplorasi hubungan bisnis tertentu dengan kejahatan. Meskipun teori mungkin menyatakan bahwa jenis bisnis tertentu (misalnya, bar) akan dikaitkan dengan peningkatan kejahatan, hubungan ini dengan kejahatan mungkin berbeda tergantung pada konteks terdekat dari jumlah bisnis, campuran bisnis, jumlah dan karakteristik penduduk lingkungan, atau fitur lain dari lingkungan yang dibangun dan sosial (yaitu, konteks ekologi). Meskipun demikian, penelitian sering kali tidak memperhitungkan konteks ekologi ini, meskipun kemungkinan besar hal itu penting.
Tantangannya adalah bahwa studi yang berfokus pada jenis bisnis tertentu—misalnya, bar secara khusus (Gorman et al., 2001 ; Roncek & Maier, 1991 ) atau tempat penjualan minuman keras secara lebih luas (Feng et al., 2018 ; Scribner et al., 1999 )—hanya akan mengukur keberadaan tempat penjualan minuman keras ini dan menilai apakah tempat penjualan minuman keras ini terkait dengan tingkat kejahatan. Keterbatasan dari strategi ini adalah bahwa setiap bar tertentu terletak dalam konteks tertentu yang tidak diperhitungkan—yaitu, seperti apa tampilan blok lainnya. Masalah ini juga muncul untuk studi yang menanyakan apakah karakteristik bisnis tertentu memengaruhi kejahatan (Linning & Eck, 2021 ). Sejauh mana konteks ekologis terkait bisnis ini penting biasanya tidak dipertimbangkan. Meskipun bisnis tertentu dapat memengaruhi daya tarik pelanggar atau target ke suatu lokasi, bisnis lain di blok jalan tersebut juga dapat memengaruhi campuran pelanggar dan target di jalan tersebut dan, oleh karena itu, jumlah kejahatan.
Meskipun beberapa penelitian memang telah mempertimbangkan bagaimana fitur konteks bisnis dapat memengaruhi kejahatan di blok jalan, penelitian ini masih terbatas cakupannya. Misalnya, strategi yang digunakan adalah mengukur beberapa jenis tempat usaha dan menilai apakah tempat usaha tersebut terkait dengan tingkat kejahatan (Smith et al., 2000 ; Wilcox et al., 2004 ). Namun, strategi seperti itu sering kali tidak memperhitungkan fitur lain dari blok tempat bisnis berada, seperti jumlah bisnis yang ada di sana, campuran bisnis tersebut, atau populasi penduduk di blok tersebut dan area sekitarnya. Faktor-faktor ini dapat membuat blok lebih ramai, yang dapat menarik lebih banyak target potensial. Faktor-faktor ini juga dapat membuat jalan lebih menarik untuk diamati oleh penduduk, yang akan meningkatkan potensi penjaga melalui “mata di jalan” ini (Jacobs, 1961 ). Dengan demikian, mempertimbangkan konteks ini, serta dampak yang berpotensi berbeda terhadap kejahatan dari berbagai jenis bisnis, merupakan tantangan metodologis dan teoritis yang masih dihadapi literatur. Memang, penelitian jarang mempertimbangkan apakah campuran bisnis di suatu blok memengaruhi tingkat kejahatan.
Dalam studi ini, kami mengeksplorasi pertanyaan-pertanyaan ini menggunakan kumpulan data besar blok di California Selatan. Di bagian berikutnya, setelah mempertimbangkan secara singkat alasan teoritis untuk mengharapkan hubungan antara bisnis dan kejahatan, kami membahas pertimbangan tentang cara mengukur konteks bisnis saat mengeksplorasi pertanyaan umum ini. Kami kemudian dapat menjelaskan apa yang telah dilakukan studi yang ada dan asumsi strategi pemodelannya. 1 Pertimbangan ini menginformasikan model kami, dan kami memperhitungkan konteks bisnis di suatu blok sambil menilai apakah jenis bisnis tertentu lebih kuat terkait dengan kejahatan lokal. Kontribusi penting adalah memperhitungkan campuran bisnis di suatu blok dan bagaimana mereka memengaruhi tingkat kejahatan. Kami juga memperhitungkan kemungkinan endogenitas di mana kejahatan memengaruhi bisnis dengan memperkirakan model variabel instrumental.
2 TINJAUAN PUSTAKA
2.1 Alasan teoritis mengapa bisnis dapat memengaruhi kejahatan
Berbagai teori menyatakan adanya hubungan antara lingkungan bisnis dan kejahatan. Salah satu perspektif adalah bahwa hubungan positif linear akan ada antara jenis bisnis tertentu dan kejahatan. Banyak teori yang menyatakan adanya hubungan seperti itu. Misalnya, dalam teori aktivitas rutin, bisnis menarik target potensial dan pelaku kejahatan, yang dapat mengakibatkan lebih banyak peluang kejahatan. Demikian pula, teori pola kejahatan menyatakan bahwa generator kejahatan adalah bisnis yang menarik banyak pelanggan—target potensial—yang sekali lagi akan mempertemukan pelaku potensial dan target. Implikasinya adalah bahwa setiap bisnis yang berhadapan dengan konsumen—bisnis yang secara langsung melayani pelanggan—akan berhubungan positif dengan tingkat kejahatan.
Teori pola kejahatan lebih jauh menekankan bahwa beberapa bisnis akan secara tidak proporsional lebih menarik bagi pelanggar karena mereka memiliki target yang lebih menarik dan cocok. Misalnya, bisnis yang menyediakan minuman keras seperti bar akan memiliki pelanggan yang meninggalkan mereka yang mungkin agak tidak berdaya karena mabuk, menjadikan mereka target yang lebih menarik (Parker, 1995 ). Sebagai contoh lain, beberapa bisnis menarik klien yang membawa uang tunai, seperti toko ganja di komunitas yang tidak mengizinkan penggunaan kredit (Contreras, 2016 ), atau tempat usaha perbankan pinggiran seperti pencairan cek atau pegadaian (Kubrin & Hipp, 2016 )—di mana pelanggan menjadi target perampokan yang menarik. Oleh karena itu, tempat usaha tertentu secara khusus dapat meningkatkan beberapa peluang kejahatan.
Sebuah komplikasi adalah bahwa teori aktivitas rutin memprediksi hubungan nonlinier antara bisnis dan kejahatan. Yaitu, meskipun bisnis dapat menarik pelanggar dan target—yang diharapkan dapat meningkatkan kejahatan—mereka juga dapat menumbuhkan wali yang lebih cakap di lingkungan tersebut, yang diharapkan dapat mengurangi kejahatan. Wali ini dapat menjadi pelanggan di area yang tertarik dengan lingkungan tersebut. Mereka memiliki potensi untuk tidak hanya menjadi wali aktif dengan menelepon polisi atau melakukan intervensi langsung tetapi juga menjadi wali pasif mengingat potensi mereka untuk bertindak sebagai saksi jika ada pelanggar yang memilih untuk melakukan kejahatan. Dengan demikian, kehadiran mereka yang lebih besar mungkin akan berdampak negatif pada kejahatan, yang dikombinasikan dengan efek positif awal akan menyiratkan hubungan positif yang melambat dengan kejahatan yang bahkan mungkin berubah menjadi negatif di beberapa titik (Birks & Davies, 2017 ). Lebih jauh lagi, keramaian jalan-jalan tersebut membuatnya lebih menarik untuk diamati oleh penduduk dan pemilik toko, meningkatkan kemampuan kontrol sosial, yang dijelaskan oleh Jane Jacobs ( 1961 ) sebagai “mata di jalan.” Memang, sebuah studi mengukur keberadaan bisnis lokal di suatu blok dengan asumsi bahwa pemiliknya akan lebih cenderung memberikan kontrol sosial (Kim & Hipp, 2022 ). Implikasinya adalah kita perlu mengukur konteks bisnis di suatu blok, terutama bisnis yang berhadapan langsung dengan konsumen.
2.2 Ekologi bisnis dan kejahatan
Seperti yang dijelaskan, beberapa teori menyatakan bahwa berbagai fitur lingkungan bisnis akan memengaruhi tingkat kejahatan. Teori-teori ini berpendapat bahwa bisnis dapat memengaruhi jumlah pelanggar, target, atau bahkan wali di suatu lokasi. Berbagai prediksi teoritis ini menyiratkan bahwa pengujian hubungan yang mungkin ini cukup rumit. Di bagian ini, kami mempertimbangkan tantangan-tantangan ini untuk menyoroti asumsi-asumsi yang sering muncul dalam penelitian yang ada. Kami menyediakan model-model formal tersirat dalam lampiran teknis di akhir artikel ini.
Salah satu strategi adalah dengan memasukkan ukuran jumlah total bisnis dalam analisis (Steenbeek et al., 2012 ). Dalam kasus ini, semua jenis bisnis di satu lokasi dijumlahkan menjadi satu ukuran tunggal. Perhatikan bahwa asumsi model ini adalah bahwa semua bisnis berdampak sama terhadap jumlah insiden kejahatan, dan oleh karena itu, setiap bisnis tambahan (jenis apa pun) akan meningkatkan insiden kejahatan (dengan asumsi koefisien positif).
Di sisi ekstrem lainnya adalah para peneliti yang berfokus pada satu jenis tempat usaha dan bagaimana hal itu terkait dengan kejahatan. Misalnya, sebuah badan penelitian telah mempelajari hubungan antara bar di lingkungan dan insiden kejahatan (Gorman et al., 2001 ; Roncek & Maier, 1991 ). Perhatikan bahwa strategi pemodelan ini sering membuat hitungan jumlah bar (atau bisnis lain yang menarik) tetapi tidak memperhitungkan jenis bisnis lain di lingkungan tersebut. Dalam hal ini, asumsinya adalah bahwa bisnis lain tidak memiliki dampak pada kejahatan, yang kemungkinan merupakan asumsi yang kuat dan tidak dapat dipertahankan. Asumsi ini dapat dilonggarkan dengan memasukkan ukuran total bisnis (dikurangi yang menarik) dalam model juga, meskipun hal ini jarang dilakukan. Model alternatif ini tidak lagi mengasumsikan bahwa bisnis lain tidak berdampak pada kejahatan, tetapi mengasumsikan bahwa semua jenis bisnis lain berdampak serupa pada kejahatan.
Strategi yang menyeluruh adalah membuat ukuran semua jenis bisnis yang ada di lingkungan dan memasukkan ukuran-ukuran ini ke dalam model. Pendekatan ini akan melonggarkan asumsi bahwa semua jenis bisnis memiliki dampak yang sama terhadap kejahatan, dan setiap jenis bisnis akan diizinkan untuk memiliki dampak terpisah terhadap kejahatan. Misalnya, studi sesekali telah mengadopsi varian dari strategi ini dengan memasukkan ukuran banyak jenis usaha (Bernasco & Block, 2011 ) atau dengan memasukkan ukuran banyak jenis penggunaan lahan (Stucky & Ottensmann, 2009 ). Meskipun demikian, banyak jenis bisnis lain tidak termasuk dalam model. Kelemahan lebih lanjut dari strategi ini adalah bahwa ia memperkirakan banyak koefisien—dan bahkan lebih banyak lagi, jika bisnis dikategorikan lebih halus. Jika jumlah jenis bisnis dapat dikurangi, model ini dapat lebih mudah diperkirakan. Perhatikan bahwa jenis bisnis yang diagregasi ke dalam setiap kategori yang lebih luas harus memiliki hubungan yang sama dengan kejahatan—yaitu, koefisien yang sama dari model yang memuat semua jenis bisnis sebagai ukuran terpisah—karena model agregat akan memperkirakan satu koefisien untuk setiap agregasi jenis bisnis. 2 Meskipun demikian, diperlukan lebih banyak panduan teoritis untuk kategorisasi tersebut. Lebih jauh, strategi ini tidak memperhitungkan potensi hubungan nonlinier antara bisnis dan kejahatan, dan mengasumsikan bahwa setiap jenis bisnis memiliki dampak yang independen dari bisnis lain, isu yang akan kita bahas nanti.
2.3 Kategori bisnis
Melonggarkan asumsi bahwa semua bisnis memiliki dampak yang sama terhadap kejahatan menimbulkan tantangan tentang cara mengklasifikasikan bisnis ke dalam kategori yang berbeda berdasarkan hubungan yang diharapkan dengan kejahatan. Hanya panduan teoritis terbatas yang tersedia untuk klasifikasi tersebut. Misalnya, satu strategi umum dalam literatur membedakan antara bisnis yang berhadapan dengan konsumen dan yang tidak berhadapan dengan konsumen (Hipp & Luo, 2022 ). Perbedaan ini wajar untuk dibuat terkait dampak pada kejahatan karena bisnis yang berhadapan dengan konsumen menurut definisi menarik pelanggan dan, oleh karena itu, biasanya memberikan lebih banyak peluang kejahatan. Bisnis yang tidak berhadapan dengan konsumen (atau yang tidak berhadapan dengan konsumen) (seperti gedung perkantoran untuk pekerja kerah putih atau pabrik untuk pekerja kerah biru) tidak menarik pelanggan dan, oleh karena itu, memberikan lebih sedikit peluang kejahatan. Lebih jauh lagi, bisnis yang terakhir ini menyediakan pekerjaan bagi penduduk lokal dan, oleh karena itu, bahkan dapat mengurangi jumlah pelanggar potensial di suatu area, yang akan mengurangi kejahatan di area umum (Hipp & Luo, 2022 ). Dengan demikian, dampak yang diharapkan terhadap kejahatan akan berbeda dari bisnis yang berhadapan dengan konsumen.
Bisnis yang berhadapan dengan konsumen ini dapat dipilah lebih lanjut berdasarkan kecenderungan yang diharapkan untuk menjadi generator kejahatan karena menarik pelanggan (dan karenanya menjadi target) atau menjadi penarik kejahatan yang secara tidak proporsional menarik pelanggar (Brantingham & Brantingham, 1995 ). Sebuah strategi yang digunakan oleh satu studi mencakup kategori karyawan ritel dan makanan, selain total karyawan, pada segmen jalan (Hipp et al., 2021 ). Disagregasi lebih lanjut juga dimungkinkan berdasarkan apakah tempat usaha tertentu diharapkan menjadi fasilitas berisiko berdasarkan berbagai fitur idiosinkratik tempat usaha tersebut, meskipun kami tidak membahasnya lebih lanjut karena kesulitan pengukuran (Bowers, 2014 ).
Selain itu, bisnis nonkonsumen dapat dibagi menjadi beberapa subkategori untuk bisnis yang diperkirakan berdampak berbeda terhadap kejahatan. Misalnya, studi yang mengukur penggunaan lahan di lokasi mikro telah membedakan antara penggunaan lahan kantor dan pabrik (Boessen & Hipp, 2015 ; Hipp et al., 2021 ; Kubrin & Hipp, 2016 ). Dengan bisnis, perbedaan juga dapat dibuat antara bisnis kerah putih dan kerah biru. Mengingat bahwa taman kantor dan area pabrik dapat menjadi lingkungan sosial yang sangat berbeda, kita mungkin berharap keduanya memiliki hubungan yang berbeda dengan kejahatan. Meskipun demikian, meskipun beberapa penelitian telah membuat perbedaan ini menggunakan kategori penggunaan lahan yang luas, sedikit penelitian yang membuat perbedaan ini menggunakan data pada bisnis tertentu.
Bisnis yang berhadapan dengan konsumen ini dapat dipilah lebih lanjut berdasarkan kecenderungan yang diharapkan untuk menjadi generator kejahatan karena menarik pelanggan (dan karenanya menjadi target) atau menjadi penarik kejahatan yang secara tidak proporsional menarik pelanggar (Brantingham & Brantingham, 1995 ). Sebuah strategi yang digunakan oleh satu studi mencakup kategori karyawan ritel dan makanan, selain total karyawan, pada segmen jalan (Hipp et al., 2021 ). Disagregasi lebih lanjut juga dimungkinkan berdasarkan apakah tempat usaha tertentu diharapkan menjadi fasilitas berisiko berdasarkan berbagai fitur idiosinkratik tempat usaha tersebut, meskipun kami tidak membahasnya lebih lanjut karena kesulitan pengukuran (Bowers, 2014 ).
2.4 Apakah hubungan antara bisnis dan kejahatan nonlinier?
Meskipun badan penelitian telah mengasumsikan hubungan positif linear antara bisnis dan kejahatan, asumsi ini mengandaikan bahwa bisnis hanya menyediakan target bagi pelanggar. Komplikasinya adalah bahwa bisnis sering menarik pelanggan yang dapat, pada konsentrasi tinggi, berfungsi sebagai penjaga di lingkungan tersebut, yang menyiratkan hubungan nonlinier antara bisnis dan kejahatan. Misalnya, satu studi menggambarkan hubungan nonlinier antara bisnis dan gangguan bahkan ketika memperhitungkan karakteristik sosiodemografi lingkungan (Steenbeek et al., 2012 ). Sebuah studi blok di empat kota menunjukkan hubungan nonlinier yang kuat antara bisnis dan perampokan (Hipp et al., 2021 ), dan penelitian menemukan hubungan nonlinier antara area komersial dan kejahatan kekerasan di Columbus, Ohio (Browning et al., 2010 ). Implikasinya adalah perlunya memperhitungkan kemungkinan hubungan nonlinier antara bisnis dan kejahatan dengan, misalnya, termasuk istilah kuadrat bisnis. Harapannya adalah karena kehadiran lebih banyak wali, hubungan positif yang melambat secara keseluruhan akan ada antara total bisnis dan kejahatan atau bahkan mungkin berubah menjadi negatif pada konsentrasi tinggi karena jumlah wali yang tinggi mengalahkan efek peluang dari lebih banyak bisnis. Meskipun efek nonlinier bisnis tersebut mudah untuk ditentukan dan diperkirakan, sangat sedikit penelitian yang mempertimbangkannya (pengecualian termasuk Browning et al., 2010 ; Hipp et al., 2021 ; Kim & Hipp, 2022 ).
Whereas only a few studies have considered a possible nonlinear relationship between businesses and crime, almost none have done so while considering the effects of specific types of business establishments on crime. Examples of strategies testing the impact of multiple business types typically fail to account for total businesses. For example, one study measured five different types of facilities (Groff & Lockwood, 2014) but did not include a measure of total businesses, and it further noted the need for research on additional facilities. Another example was a study measuring 14 types of facilities and their relationship to robberies across three cities, but the study also did not account for total businesses (Barnum et al., 2017). Yet another study focused on how the context of the physical environment might impact crime; even though this study measured nine types of establishments in the area, it also did not account for total businesses and therefore did not account for potential nonlinearity (Wilcox et al., 2004).
2.5 Does the relationship between a business type and crime depend on the context?
Another aspect of the business context, besides the total number of businesses, is the mixture of businesses in an environment. Nonetheless, expectations conflict on how such mixing would impact crime. One perspective was that of Jacobs (1961), who argued that a mixture of businesses on a street would enhance the vibrancy of an area, resulting in more people on the street. She posited that this would reduce crime by bringing about more social control through eyes on the street. If a street, however, is more vibrant and attracts more people, then it would not only increase the number of possible guardians. In fact, it would also increase the number of potential targets and offenders, which could increase crime (Birks & Davies, 2017). A further complication is that Jacobs (1961) posited that such business mixing would bring about more consistency in the number of people on the street throughout the hours of the day, given that different businesses might have different peak hours of attracting customers. Although Jacobs posited that “dead” times with few people about, which she assumed to be the highest crime-risk time periods, would be reduced, we do not know what the nonlinear relationship between ambient population and crime at a point in time looks like (Hipp, 2016).
The challenge is that at least two different possible nonlinear models might capture the relationship between ambient population and crime: (1) an inverted-U relationship or (2) a slowing positive relationship. Jacobs (1961) implicitly posited an inverted-U relationship, in which crime incidents are reduced when the ambient population is large enough. The empirical evidence for an inverted-U relationship, however, is mixed. One study found evidence of an inverted-U relationship during some hours in the city of Santa Ana, California (Hipp, 2016). Other evidence, however, suggests a slowing positive relationship as found in one empirical study (Hipp et al., 2019) and in a simulation study (Birks & Davies, 2017). Note that a slowing positive relationship for crime incidents implies that the risk (or rate) of crime is lower given that the ambient population is increasing at a linear rate. This lower relative risk might make it feel safer to some people even though the number of crimes is not decreasing (for a nice discussion of conceptualizing relative risk, see Cohen & Felson, 1979).
The consequence of these two possible nonlinear relationships is that Jacobs’s (1961) conclusion that evening out the ambient population over the hours of the day will always reduce crime is ambiguous. If it is an inverted-U relationship in which crime levels decrease at higher ambient population levels, then whether evening out the number of people on a street reduces crime would depend on the absolute number of people throughout the day rather than on just the relative distribution across the hours of the day. What is needed is that the ambient population is consistently high and, therefore, on the right side of the graph with a diminishing crime level. If instead the ambient population is consistently at the midlevel, this would be the peak inflection point, and it would not reduce crime at all. So, this consistently increased activity needs to exceed some threshold to have a negative effect. If it is in fact a diminishing positive relationship, then evening out the number of people on a street across time periods cannot reduce the level of crime. The implication is that if business mixing indeed increases the vibrancy of a street, it is unclear whether this will exhibit a positive or negative relationship with crime, and it might depend on the number of businesses.
Whereas Jacobs (1961) focused on the potential for guardians in the environment, a second perspective is that business heterogeneity might impact the mixture of offenders. Note that tests of the impact of businesses on local crime ignore the potential role of offenders. The focus instead is on how businesses may provide suitable targets, and sometimes on how they can provide potential guardianship, but offenders are typically implicit in these studies and not measured. Although crime pattern theory discusses the role of offenders and how they might move about the spatial landscape as determined by the environmental backcloth, the empirical ecology of crime studies rarely takes this into account (Brantingham & Brantingham, 2016).
In our context here, business heterogeneity may not simply increase the ambient population at a location overall, or consistently over the hours of the day as hypothesized by Jacobs (1961), but might impact the mixture of offenders coming to a location (van Sleeuwen et al., 2021). The consequence, however, might be an increase in crime rather than a decrease as posited by Jacobs (1961). For example, the variety of businesses at a location could provide a variety of criminal opportunities, increasing the flow of offenders there (Song et al., 2019). The variety of businesses would potentially increase the number of potential offenders for whom this will become a frequent activity node (Brantingham & Brantingham, 2016). Some evidence does show that co-offenders potentially meet each other through activity nodes (Rowan et al., 2022), and a wide variety of businesses may enhance this. This business mixing may also make the location attractive to a wider variety of offenders, increasing crime incidents (Ruiter, 2017). As another potential mechanism, a location with a wider variety of businesses may attract a wider variety of persons to the location, allowing offenders to blend into the environment better. Therefore, the location could become a more attractive target, again increasing crime incidents. Furthermore, if this business mixing is combined with more businesses, we would expect the location to be even more attractive to offenders. This possibility can be tested by including an interaction term between business heterogeneity and the number of businesses, with the expectation that this mixing may impact crime differently when more businesses are in the environment.
Despite these possible impacts of business heterogeneity on crime, we are aware of no studies addressing this question. One strategy adopted by some studies is to create a measure of land-use mixing, although these categories are typically much broader than would be used to capture business mixing. For example, one study created a land-use mixing measure based on eight broad categories of land uses and found a positive relationship between robbery and burglary (Wo, 2019). Another study measured the mixing of five main types of land use and found that street segments with higher levels of mixing experienced more crime (Kim & Hipp, 2021). On the other hand, a study using the larger geographic units of block groups found that mixed land use was associated with lower property crime rates, even when accounting for specific business types (Zahnow, 2018).
2.6 The context of the residential population for businesses
A final component of the local context that might impact the relationship between businesses and crime is the size of the residential population. At one extreme, some business districts are located separately from residential areas and, therefore, have no residential population. At the other extreme is a business located on an otherwise residential street. In between are mixed-use streets that contain both numerous businesses and a sizable residential population. Indeed, this latter category is what Jacobs (1961) was referring to in her book, as she lived in the mixed-use Greenwich Village area of New York City. In Jacobs’s view, the residential population may have important consequences in a vibrant business location as the mixing of businesses could make the street more interesting to watch, which will increase the “eyes on the street” and therefore the social control capability. Furthermore, the residential population knows who does or does not belong, and therefore, it is expected to have different consequences for crime opportunities compared with the ambient population, which includes nonresidents. The implication is a reduction in crime in such instances if the residential population is sizable enough.
Some scholars also speculate that the sociodemographic characteristics of residents may have important consequences for how businesses are related to crime. This speculation comes from the literature attempting to link routine activity theory with social disorganization theory (Smith et al., 2000; Wilcox et al., 2003, 2012). In these studies, a common strategy is to assess whether the level of concentrated disadvantage in the neighborhood moderates the possible opportunity effects that occur with businesses or land use (Hipp et al., 2022; Smith et al., 2000; Tillyer et al., 2020). We will consider these possibilities in our analyses.
3 DATA AND METHODOLOGY
3.1 Data
The study site for our analyses was Southern California. We combined data collected from police agencies with sociodemographic data from the U.S. Census and business data from the Reference USA Historical Business Dataset. To capture the micro-spatial location of crime, we aggregated the crime data into blocks. A challenge with micro-level studies is that many small units can have no population; in which case, the sociodemographic variables are undefined. Thus, a large amount of systematic missingness can result if the researcher simply excludes such units. We addressed this issue by using multiple imputations—using five imputations and then combining the results based on Rubin’s strategy (Rubin, 1976). We then avoided excluding these observations from the study.
3.2 Dependent variables
The crime data come from the Southern California Crime Study (SCCS). In the SCCS, researchers contacted each police agency in Southern California3 and requested address-level incident crime data for the years 2005–2012. Many agencies willingly shared their data. The subsequent crime data cover 83.3% of the region’s population. The data come from crime reports officially coded and reported by the police departments.4 The SCCS classified crime events into six Uniform Crime Reporting (UCR) Program categories: homicide, aggravated assault, robbery, burglary, motor vehicle theft, and larceny. In this study, we averaged crime events from 2009 to 2011 to smooth over year-to-year fluctuations. Crime events were geocoded for each city separately to latitude–longitude point locations using ArcGIS 10.2 and subsequently aggregated into blocks. The average geocoding match rate was 97.2% across the cities, with the lowest value at 91.4%.5 These data have been used in several studies (Kubrin & Hipp, 2016; Kubrin et al., 2016). We did not use homicides as they were too rare for statistical analyses in these small geographic units.
3.3 Independent variables
Our key independent variables capture the presence of businesses on blocks. We geocoded each business and placed it in its appropriate block. One challenge when measuring businesses is accounting for different-sized businesses. One strategy simply sums the number of businesses on a block, which ignores different business sizes. Another strategy sums the number of employees in firms on a block, which does not distinguish between, for example, one business with 200 employees versus 20 businesses with 10 employees each. Our solution was to create a novel measure of the logged employees (we added one before logging and then one after logging) in each business on a block and then sum these logged counts. Adding one before logging accounts for firms with no employees and adding one after logging results in a firm with no employees (a sole proprietor) having a value of 1, but larger firms will be weighted more heavily (but not as much as if simply counting the number of employees instead of logged employees). Summing this for all businesses on a block gives us a measure of total businesses. We also created a squared version of this measure and all others described here to test for nonlinearity.
To classify types of businesses, we used the six-digit North American Industry Classification System (NAICS) code. First, we determined consumer-facing businesses based on a classification system used by Kane et al. (2017) that assesses whether the business primarily serves the public as customers (see Kane et al. for a complete description of the classification codes). This classification identifies 31 classes of consumer-facing businesses.6 We created a measure of business heterogeneity as a Herfindahl index of these 31 business types; this is computed as one minus a sum of squares of the proportions of each of these types. The Herfindahl index (also known as the “Simpson index”) has a long history of being used as a measure of mixing (Simpson, 1948). We created a measure of nonconsumer businesses by subtracting the consumer-facing businesses from the total businesses. We further divided these nonconsumer businesses into white-collar businesses (e.g., office-based) and blue-collar businesses (e.g., factories and light industry) based on the two-digit NAICS code.7 We tested this measure given that these two classes of jobs imply different environmental settings that might impact crime levels, and empirical evidence from studies using land-use characteristics supports this approach. For example, a study in Indianapolis, Indiana, found that stronger positive relationships existed with crime in white-collar versus blue-collar locations (Stucky & Ottensmann, 2009). Likewise, a study of blocks across seven cities found a stronger positive relationship for office buildings compared with industrial buildings, especially for robbery and larceny (Boessen & Hipp, 2015).
To test whether specific types of businesses matter, 19 business categories were determined by grouping together businesses that provide similar goods or services to consumers (details can be found in Table 1). For instance, gas stations, auto services, and repair services were categorized into repair and auto services, whereas full-service restaurants, limited-service food and beverage businesses, drinking places, and movie theaters were categorized into food, drinks, and entertainment.
TABLE 1. Summary statistics of variables used in analyses.
All Blocks Blocks with Businesses
Variable Mean SD Mean SD
Dependent variables
Aggravated assault .22 .74 .34 .92
Robbery .13 .51 .23 .68
Burglary .45 1.15 .72 1.49
Motor vehicle theft .32 .89 .50 1.15
Larceny 1.13 5.00 1.89 6.74
Businesses
Total businesses (/ 100) .07 .30 .14 .41
Business heterogeneity .11 .25 .20 .31
Nonconsumer businesses (/ 100) .02 .17 .05 .23
White-collar businesses (/ 100) .02 .14 .04 .19
Blue-collar businesses (/ 100) .01 .06 .02 .08
Individual business types
Food, drinks, and entertainment .60 3.12 1.19 4.30 Full-service restaurants, limit-service food and beverage businesses, drinking (alcohol) places, and movie theaters
Beer and convenience stores .05 .37 .10 .51 Convenience stores and beer, wine, and liquor stores
Retail .81 5.65 1.59 7.85 General merchandise, home products, personal products, specialty, and apparel retailing
Necessity products and services .13 .80 .25 1.11 Grocery and drug stores
Specialty food .09 .61 .17 .84 Specialty food
Personal and household services .41 1.98 .81 2.72 Hair care services, laundry, and other personal services
Financial services .27 1.79 .54 2.49 Deposit-taking institutions and personal financial businesses
Child care and child learning .22 1.18 .42 1.63 Child care services and elementary and secondary schools
Recreation and learning .10 .69 .20 .95 Recreational facilities and instruction and other learning facilities
Community organizations .33 1.83 .65 2.53 Religious and social service organizations
Medical and health 1.24 19.14 2.44 26.78 Medical laboratories, hospitals, and healthcare provider offices
Repair and auto services .43 2.06 .85 2.83 Gas stations, auto services, and repair services
Administration, management, and utilities .39 2.32 .76 3.20 Utilities, public administration, management of companies and enterprises, administrative and support, waste management, and remediation services
Manufacturing .34 2.23 .67 3.09 Manufacturing
Construction .39 1.81 .76 2.49 Construction
Transit .00 .07 .00 .10 Transit
Wholesale trade, transport, and warehousing .53 3.45 1.04 4.78 Wholesale trade, transport, and warehousing
Resource acquisition businesses .02 .21 .03 .29 Agriculture, forestry, fishing, and hunting and mining, quarrying, and oil and gas extraction
Control variables
Concentrated disadvantage −.31 1.05 −.26 1.05
Residential stability 10.82 5.32 10.52 5.26
Racial/ethnic heterogeneity .36 .22 .40 .21
Percent Black 6.80 13.03 6.82 13.56
Percent Latino 34.54 30.09 35.24 29.55
Percent Asian 10.69 15.55 10.87 15.29
Percent vacant units 9.61 13.64 7.88 11.41
Percent aged 16–29 18.90 11.71 20.08 10.98
Population (logged) 3.06 2.12 3.87 1.90
Population within 2.5 miles (exponential decay) 26.39 19.54 28.89 20.64
Tweets (logged) .86 1.39 1.30 1.64
Note. N = 187,714 blocks.
3.4 Control variables
To minimize the possibility of spurious effects, we also included a set of variables shown to be important in the ecology of crime literature. Specifically, we included several sociodemographic characteristics based on data collected from the 2010 U.S. Census and the American Community Survey (ACS) five-year estimates for 2008–2012. For variables that the Census only provides in larger units, such as block groups or tracts, we imputed them to blocks. We accomplished this imputation using the ecological inference approach that imputes them based on other characteristics of the block, an approach that Boessen and Hipp (2015) showed is typically preferred to a simple areal imputation.8
We constructed a measure of concentrated disadvantage based on a factor score from a principal components analysis of percent at or below 125% of the poverty level, average household income, percent with at least a bachelor’s degree, and percent single-parent households. We measured residential stability as a factor score based on a confirmatory factor analysis of percent owners, percent in the same house 1 year ago, and the average length of residence. We captured the racial/ethnic composition with percent Black, percent Asian, and percent Latino (with percent White and Other as the remaining category). We constructed a measure of racial/ethnic heterogeneity based on the Herfindahl index combining these same five racial/ethnic categories as a sum of squared proportions, subtracted from 1. To account for vacant units, we computed the percent vacant units. The possible presence of offenders is captured with the percent aged 16–29. Although this measure is not ideal given that it conflates attached and nonattached youth—that is, those engaged in education or the labor force, as opposed to those who are not (McCall et al., 2013)—we included this as one possible proxy for potential offenders. We also included measures of the general population, under the presumption that anyone could be an offender, and therefore, we computed the population (logged after adding 1) of the block (and a squared version), and captured the population in the surrounding area as the population within 2.5 miles based on an exponential decay function (β = –1). This decay function captures potential offenders in the surrounding area.
One possibility is that our business measures are simply proxies for the presence of the ambient population. To account for this possibility, we also included a measure of the number of geocoded tweets in the block. These data were collected by researchers using the Twitter Streaming API over an eight-month period (from May 2015 to December 2015; Hipp et al., 2019). Only tweets with geolocation coordinates (GPS) within the geographic boundaries of Southern California were collected, which allowed for collecting all available geolocated tweets within Southern California during the time period. We determined each unique tweet by a user (based on ID variable) in a census block during a specific hour of the day—thus, multiple tweets by a user at a point in time were not counted. Therefore, we established a count of the number of persons in a block during an hour. We totaled these up over the time period for each block and then log transformed this measure given the strong skew it exhibited as some locations had a high number of tweets. We used this result as a proxy of the ambient population.9
The summary statistics for the variables used in the analyses are shown in Table 1. The first two columns show the summary statistics for the entire sample, whereas the last two columns show them for the subset of blocks that have at least one business on them. We see that 50%–67% more crime incidents tend to occur on blocks that have some businesses compared with the full sample of blocks. For example, the average block in the sample has 0.14 robbery incidents, whereas the average block with businesses has 0.23. Businesses appear to be equally split between consumer-facing and nonconsumer businesses; however, more white-collar businesses exist than blue-collar ones. Among the specific types of businesses, the most frequent are medical and health, retail, wholesale trade, and food, drinks, and entertainment.
3.5 Methodology
The endogeneity of our business measures needed further examination. Therefore, we estimated instrumental variable models to account for this endogeneity; as instruments, we used the same business measures in the year 2000 under the presumption that the business composition in 2000 would causally impact the business composition in 2010 but would have no direct additional impact on crime levels beyond the impact that businesses in 2010 have. Therefore, the first-stage equations regress each of our business variables of interest on the same business measures in 2000, as well as all variables in the model—these are the reduced form equations. We obtained the predicted values of our business measures from these first-stage equations, and these predicted values were then included in the second-stage equations—that is, our final estimated models.
Given that the outcome variables are crime counts with overdispersion, we estimated negative binomial regression models for the five crime types. These models were estimated as follows:
(1)
where y is the count of crime events in the block, contains the predicted values of the various business variables from the first-stage equations, XS contains the various sociodemographic variables, В1 and В2 are vectors of parameters capturing the relationship between these measures and the crime count, and μ is a parameter capturing the overdispersion based on a gamma distribution. We estimated a series of models, all of which include our control variables. The first model includes the total number of businesses (linearly) and business heterogeneity. The second model tests for a nonlinear relationship between total businesses and crime. Model 3 splits businesses into consumer-facing businesses and nonconsumer businesses. Model 4 further splits nonconsumer businesses into white- and blue-collar businesses. Model 5 adds our 19 business types to model 4. Our final models test for interactions between the business heterogeneity and total business measures with our two measures of population: population in the block and population in the surrounding 2.5-mile buffer, as well as block concentrated disadvantage.
4 RESULTS
4.1 Aggravated assault models
We next turn to the initial aggravated assault models, presented in Table 2. In model 1, we included the measure of total businesses and the business heterogeneity measure (along with the full set of control variables). We found that both measures were positively associated with aggravated assaults and that the relationship for business heterogeneity was particularly strong. A 1 standard deviation increase in business heterogeneity was associated with 50% more aggravated assaults (exp (1.36 × 0.3) = 1.50). Thus, we found no evidence that business heterogeneity reduces crime, as hypothesized by Jacobs (1961). A positive relationship also exists for total businesses, although this effect is smaller than business heterogeneity as a 1 standard deviation increase is associated with 6% more aggravated assaults. Earlier we noted that a multiplicative relationship may exist between the total number of businesses and business heterogeneity, but we found no such evidence when we tested this effect for all crime types.10
TABLE 2. Aggravated assault instrumental variable models: Various business measures.
Variable (1) (2) (3) (4)
Business heterogeneity 1.360** 1.218** 1.172** 1.180**
(55.49) (43.07) (41.19) (41.28)
Total businesses .142** .466** .708** 1.032**
(9.66) (13.59) (17.28) (19.70)
Total businesses squared −.068** −.103** −.115**
−(8.84) −(9.14) −(10.50)
Nonconsumer businesses −.633**
−(11.93)
Nonconsumer businesses squared .098**
(4.89)
White-collar businesses −.817**
−(9.60)
Blue-collar businesses −1.738**
−(15.18)
White-collar businesses squared .105**
(5.75)
Blue-collar businesses squared .409**
(7.90)
Intercept −2.497** −2.526** −2.524** −2.542**
−(45.40) −(45.89) −(46.09) −(46.45)
BIC 168,116 167,882 167,630 167,469
Pseudo R2 .214 .215 .216 .217
Notes. t values in parentheses. N = 187,714 blocks.
All models include control variables: concentrated disadvantage, residential stability, racial/ethnic heterogeneity, percent Black, percent Latino, percent Asian, percent vacant units, percent aged 16–29, number of tweets (logged), population (logged) and quadratic, and population within 2.5 miles.
*P < 0.05.
**P < 0.01 (two-tailed test),.
In model 2 of Table 2, we see a nonlinear relationship between total businesses and crime. This model fits better than model 1 (based on the Bayesian information criterion [BIC] value), although the improvement in pseudo R2 is modest. In model 3, we tested the nonlinear relationship between total businesses and crime while distinguishing between consumer-facing and non–consumer-facing businesses, and again, an improvement in model fit occurred (although a modest one). The coefficients for nonconsumer businesses, and squared, show the difference in nonlinearity compared with consumer-facing businesses. To provide a more intuitive sense of the size of these marginal effects, we plotted them for aggravated assault in Figure 1A. In this plot, the lowest line shows the relationship between businesses and crime in a block with all nonconsumer businesses, which is a modest positive relationship. In contrast, the top line plots the relationship between businesses and crime in a block with all consumer-facing businesses, which is a much stronger positive relationship. Also, despite the statistically significant quadratic terms in model 3, the bend of these lines is modest.
Details are in the caption following the image
FIGURE 1
Open in figure viewer
PowerPoint
Marginal relationship between businesses and crime, split by nonconsumer versus consumer-facing businesses. [Colour figure can be viewed at wileyonlinelibrary.com]
Note. N = 187,714 blocks.
In model 4 of Table 2, nonconsumer white-collar or blue-collar businesses are distinguished. A modest improvement in model fit is found based on the BIC values. Also, different coefficients exist for these two measures—showing how they differ from consumer-facing businesses—and we plotted these marginal effects in Figure 2A.11 The top line in this figure again shows that the strongest positive relationship with aggravated assaults occurs in blocks in which all the businesses are consumer facing. In contrast, the lowest line shows that in a block in which all businesses are blue collar, a notable negative relationship with aggravated assaults is found. A modest positive relationship exists with aggravated assaults in a block with all white-collar businesses (the line near the bottom).
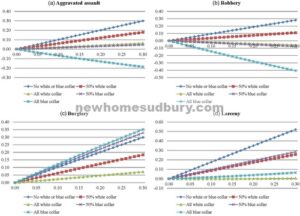
Marginal relationship between businesses and crime, based on the percentage of white- or blue-collar businesses. [Colour figure can be viewed at wileyonlinelibrary.com]
Note. n = 187,714 blocks.
4.2 Models for other four crime types
We next turn to the models for the other four crime types (Tables 3–6), and we find that the broad pattern is similar to the aggravated assault models. We continue to see a strong positive relationship between business heterogeneity and crime. A 1 standard deviation increase in business heterogeneity is associated with 35%–40% more burglaries and motor vehicle thefts, 60% more larcenies, and 95% more robberies. We also see for all of these crime types that model fit is improved when accounting for the nonlinearity of businesses and then when distinguishing between consumer-facing and non–consumer-facing businesses. The nonlinear relationship based on consumer-facing versus nonconsumer businesses across the crime types is plotted in Figure 1. Compared with Figure 1A for aggravated assault, an even sharper distinction exists between consumer-facing and nonconsumer businesses for robberies in Figure 1B. The lowest line in Figure 1B shows that a block with all nonconsumer businesses has a pronounced negative relationship with robberies. In contrast, the top line shows a strong positive relationship with robberies in blocks with all consumer-facing businesses. This finding is consistent with the expectation that more suitable targets for robberies exist when many businesses attract customers to the block. In Figure 1C, however, we see minimal difference for burglaries when distinguishing between these two categories of businesses. Burglaries typically occur when businesses are closed, and little difference is shown in the attractiveness of these two business classifications. We did not plot the results for motor vehicle thefts, but they were similar to those for burglary. The positive relationship between consumer-facing businesses and larcenies is strong in Figure 1D (the top line) and much stronger than the relationship for nonconsumer businesses (the lowest line).
TABLE 3. Robbery instrumental variable models: Various business measures.
Variable (1) (2) (3) (4)
Business heterogeneity 2.224** 2.165** 2.044** 2.083**
(78.35) (69.57) (63.91) (64.70)
Total businesses .019 .131** .580** .957**
(1.33) (4.72) (14.66) (19.59)
Total businesses squared −.014** −.070** −.074**
−(2.99) −(6.62) −(7.25)
Nonconsumer businesses −1.017**
−(23.96)
Nonconsumer businesses squared .109**
(8.24)
White-collar businesses −1.148**
−(17.27)
Blue-collar businesses −2.442**
−(20.82)
White-collar businesses squared .092**
(7.67)
Blue-collar businesses squared .522**
(10.28)
Intercept −3.642** −3.674** −3.651** −3.674**
−(61.10) −(61.24) −(59.81) −(60.55)
BIC 111,458 111,318 110,666 110,517
Pseudo R2 .277 .278 .282 .284
Notes. t values in parentheses. N = 187,714 blocks.
All models include control variables: concentrated disadvantage, residential stability, racial/ethnic heterogeneity, percent Black, percent Latino, percent Asian, percent vacant units, percent aged 16–29, number of tweets (logged), population (logged) and quadratic, and population within 2.5 miles.
*P < 0.05.
**P < 0.01 (two-tailed test),.
TABLE 4. Burglary instrumental variable models: various business measures.
Variable (1) (2) (3) (4)
Business heterogeneity 1.118** .929** .906** .889**
(58.92) (43.80) (41.87) (40.65)
Total businesses .389** .776** .880** 1.025**
(32.98) (34.26) (32.01) (29.96)
Total businesses squared −.083** −.102** −.098**
−(18.17) −(14.39) −(14.67)
Nonconsumer businesses −.276**
−(8.18)
Nonconsumer businesses squared .056**
(4.44)
White-collar businesses −.784**
−(15.55)
Blue-collar businesses .219*
(2.39)
White-collar businesses squared .095**
(10.20)
Blue-collar businesses squared −.150*
−(2.36)
Intercept −1.694** −1.708** −1.704 −1.696**
−(33.73) −(33.63) −(34.41) −(34.60)
BIC 277,668 276,849 276,666 276,408
Pseudo R2 .171 .174 .174 .175
Notes. t values in parentheses. N = 187,714 blocks.
All models include control variables: concentrated disadvantage, residential stability, racial/ethnic heterogeneity, percent Black, percent Latino, percent Asian, percent vacant units, percent aged 16–29, number of tweets (logged), population (logged) and quadratic, and population within 2.5 miles.
*P < 0.05.
**P < 0.01 (two-tailed test).
TABLE 5. Motor vehicle theft instrumental variable models: Various business measures.
Variable (1) (2) (3) (4)
Business heterogeneity .985** .715** .722** .695**
(46.19) (29.54) (29.94) (28.52)
Total businesses .340** .932** .986** 1.199**
(25.27) (32.04) (30.29) (29.83)
Total businesses squared −.133** −.133** −.135**
−(19.12) −(14.56) −(15.48)
Nonconsumer businesses −.351**
−(8.69)
Nonconsumer businesses squared .057**
(3.28)
White-collar businesses −1.143**
−(20.57)
Blue-collar businesses .459**
(4.10)
White-collar businesses squared .150**
(13.87)
Blue-collar businesses squared −.417**
−(4.71)
Intercept −2.469** −2.504** −2.495** −2.478**
−(45.54) −(45.31) −(46.32) −(46.04)
BIC 214,706 213,807 213,649 213,284
Pseudo R2 .216 .219 .220 .221
Notes. t values in parentheses. N = 187,714 blocks.
All models include control variables: concentrated disadvantage, residential stability, racial/ethnic heterogeneity, percent Black, percent Latino, percent Asian, percent vacant units, percent aged 16–29, number of tweets (logged), population (logged) and quadratic, and population within 2.5 miles.
*P < 0.05.
**P < 0.01 (two-tailed test),.
TABLE 6. Larceny instrumental variable models: Various business measures.
Variable (1) (2) (3) (4)
Business heterogeneity 1.579** 1.501** 1.387** 1.329**
(81.47) (72.77) (63.82) (60.96)
Total businesses .596** .769** 1.089** 1.783**
(38.16) (38.57) (40.23) (43.86)
Total businesses squared −.048** −.082** −.167**
−(23.29) −(12.89) −(21.88)
Nonconsumer businesses −.846**
−(21.69)
Nonconsumer businesses squared .105**
(8.23)
White-collar businesses −1.799**
−(27.16)
Blue-collar businesses −1.631**
−(19.21)
White-collar businesses squared .285**
(19.60)
Blue-collar businesses squared .385**
(9.11)
Intercept −.686** −.694** −.695** −.705**
−(9.58) −(9.55) −(9.38) −(9.66)
BIC 442,893 442,290 441,169 440,517
Pseudo R2 .159 .160 .163 .164
Notes. t values in parentheses. N = 187,714 blocks.
All models include control variables: concentrated disadvantage, residential stability, racial/ethnic heterogeneity, percent Black, percent Latino, percent Asian, percent vacant units, percent aged 16–29, number of tweets (logged), population (logged) and quadratic, and population within 2.5 miles.
*P < 0.05.
**P < 0.01 (two-tailed test).
When distinguishing between nonconsumer white- or blue-collar businesses, important distinctions must also be made, as we plotted in Figure 2. Similar to the results for aggravated assault that we described, we see in Figure 2B a similar pattern for robberies. The strongest positive relationship occurs in blocks with all consumer-facing businesses (the top line). An intense negative relationship exists, however, between the number of blue-collar businesses and robberies (the lowest line) and a flat relationship exists for white-collar businesses (the line second from the bottom). The pattern is much different for burglaries in Figure 2C and motor vehicle thefts (not plotted but similar to burglaries). Here, the strongest positive relationship with burglaries or motor vehicle thefts occurs in blocks with all blue-collar businesses, whereas the weakest relationship occurs in blocks with all white-collar businesses. So, these two broad types of nonconsumer businesses have much different relationships with these two property crimes. Finally, the other property crime of larcenies shows a weak positive relationship in blocks with all white-collar or blue-collar businesses—the lowest two lines in Figure 2D—but a strong positive relationship with consumer-facing businesses (the top line).
4.3 Separating businesses by more fine-grained categories
To this point, we have used broad categories of businesses. A more common strategy in the literature is to use more fine-grained categories—although often ignoring businesses not falling into the categories used and typically not considering nonlinearity or business heterogeneity. We initially mimicked this common strategy and estimated models with the 19 different categories we created (and the control variables), and the fits of the models were worse than nearly all models in Tables 2–6. When we instead estimated the models in which we also included the measures of total businesses and business heterogeneity, these models no longer assumed no effect from this broader business context, and a much-improved model fit occurred. The results of these latter models are shown in Table 7, and here model fit improvement is found versus those in Tables 2–6 (based on the BIC values), although the improvement in the pseudo R2 is modest (ranging from 0.005 to 0.01). Note that the coefficients for these 18 types of businesses (with one excluded) capture how their relationship differs from the nonlinear effect of total businesses.
TABLE 7. Instrumental variable models for several types of crime: Using specific categories of businesses.
Variable Aggravated Assault Robbery Burglary Motor Vehicle Theft Larceny
Business heterogeneity 1.179** 1.945** .902** .839** 1.399**
(34.17) (51.15) (34.10) (27.67) (53.20)
Total businesses .222** −.307** .556** .874** .459**
(2.65) −(3.74) (9.70) (12.39) (7.52)
Total businesses squared −.033** 0.005 −.053** −.134** −.036**
−(4.20) (.83) −(10.63) −(16.33) −(11.31)
Individual business types
Food, drinks, and entertainment .045** .029** .025** .024** .041**
(18.87) (12.49) (13.34) (11.78) (20.91)
Child care and child learning .060** .043** .051** .012** .050**
(16.85) (10.26) (18.23) (3.63) (16.83)
Retail .001 .010** .006** .009** .025**
(1.21) (9.85) (6.79) (10.11) (21.61)
Necessity products and services −.009 .092** .037** .019** .084**
−(1.22) (13.94) (6.85) (3.15) (14.87)
Recreation and learning .063** .019 .041** .023* .078**
(5.70) (1.53) (5.10) (2.42) (9.73)
Repair and auto services .011** .017** .008** .010** .015**
(3.96) (6.61) (3.91) (4.94) (6.84)
Administration, management, and utilities .017** .009** .001 .005* .014**
(6.91) (3.71) (.55) (2.20) (6.62)
Medical and health .003** .004** .000 .000 .001**
(4.30) (6.09) −(.60) (.83) (2.79)
Resource acquisition businesses −.075 −.023 .013 .027 .073*
−(1.60) −(.43) (.45) (.79) (2.40)
Manufacturing −.008* −.008* .010** −.002 −.007*
−(2.18) −(2.19) (3.39) −(.61) −(2.13)
Wholesale trade, transport, and warehousing −.001 −.001 −.005** .004* −.009**
−(.33) −(.64) −(2.75) (2.39) −(4.30)
Transit .008 .078 −.270 −.400 .191
(.03) (.36) −(1.29) −(1.95) (.98)
Financial services −.027** .017** −.004 −.011* −.009*
−(4.71) (3.19) −(1.18) −(2.51) −(2.36)
Beer and convenience stores −.010 .057** −.066** −.074** −.065**
−(.71) (4.20) −(6.01) −(6.14) −(5.86)
Construction −.021** −.032** .005 −.014** −.020**
−(4.28) −(5.63) (1.39) −(3.65) −(5.64)
Specialty food −.070** −.083** −.094** −.060** −.140**
−(4.83) −(6.10) −(8.82) −(5.23) −(13.39)
Community organizations −.011* −.014** −.030** −.032** −.034**
−(2.55) −(3.04) −(9.45) −(8.71) −(10.73)
Personal and household services −.031** −.022** −.018** −.043** −.058**
−(7.71) −(5.65) −(6.28) −(12.53) −(20.11)
BIC 166,845 109,933 275,801 212,851 438,866
Pseudo R2 .221 .289 .177 .223 .167
Notes. t values in parentheses. N = 187,714 blocks.
All models include control variables: concentrated disadvantage, residential stability, racial/ethnic heterogeneity, percent Black, percent Latino, percent Asian, percent vacant units, percent aged 16–29, number of tweets (logged), population (logged) and quadratic, and population within 2.5 miles.
*P < 0.05.
**P < 0.01 (two-tailed test).
In Table 7, we have ordered the 18 business categories from average strongest positive to strongest negative relationships with the crime types (we excluded the uncategorized category). We see when controlling for the general nonlinear relationship between businesses and crime, as well as the level of business heterogeneity, that additional food, drink, and entertainment establishments are strongly positively associated with all crime types. A 1 standard deviation increase in these establishments (weighted by logged size) is associated with 9% more burglaries to 21% more aggravated assaults. Other businesses positively associated with crime are child care and child learning, retail, necessity products, recreation and learning, repair and auto services, and administrative/management establishments. In contrast, after controlling for the nonlinear effect of total businesses, a few types are negatively associated with crime rates: personal/household services, specialty food, construction, and community organizations. Nonetheless, these associations are modest effects for these specific businesses, highlighting the importance of accounting for the general business context.
We also highlight that the models we estimated mimicking the common strategy in the literature of including measures of specific businesses without accounting for the total business context yielded biased results. For example, it is common in the literature to measure drinking establishments, and we found that the estimates for these establishments were biased upward 40%–110% in the models that did not account for the business context. Likewise, beer and liquor stores were associated with much higher levels of crime in the models not accounting for the business context, but these coefficients were dramatically smaller in the violent crime models and turned negative in the property crime models when including the business context. Several other measures were severely biased upward in the models not accounting for the business context, including child care and child learning, repair and auto services, and necessity products and services. Furthermore, whereas community organizations were positively related to crime in the models not accounting for the business context, this reversed to a negative relationship once accounting for this context, which may explain why the results are mixed for community organizations in the literature despite the expected negative relationship.
4.4 How the nearby context matters: Residential population
As one final set of tests, we assessed whether the impact of business heterogeneity is moderated by the residential population in the block, disadvantage in the block, and population in the surrounding environment.12 The block residential population captures Jacobs’s (1961) idea of potential residential guardians, whereas the population in the surrounding area represents potential offenders that might impact the level of crime near businesses. These interactions were all statistically significant. Furthermore, the model fit (based on the BIC and the pseudo R2) is superior for all of these crime types compared with the models in Table 7. The coefficients from these models are shown in Table 8, and we plotted the moderating effects of business heterogeneity and block residential population in Figure 3. The x-axis in these figures plots the residential population in the block (logged) over the range from 1 standard deviation below the mean to 1 standard deviation above the mean, and the three lines plot the expected relationship with crime in hypothetical high, average, and low business heterogeneity blocks (defined as 1 standard deviation above the mean, the mean, and 1 standard deviation below the mean). In Figure 3A, the left side of the figure shows that with no population, a large difference exists in the expected aggravated assaults between blocks with high business heterogeneity (the top line) and those with low business heterogeneity (the lowest line). As the population in the block increases, however, the importance of business heterogeneity weakens, and at the highest population levels (the right side of the figure), modest differences are found in expected aggravated assault levels based on the level of business heterogeneity. The pattern is similar for the other crime types (motor vehicle theft is similar to burglary, although not plotted here). The effect is weakest for robbery, although still present. As an alternative way to interpret these plots, a strong nonlinear positive relationship exists between block population and crime when no business heterogeneity is found, but this relationship effectively evaporates in a high business heterogeneity environment. This alternative interpretation invokes the vision of Jacobs (1961), although it does highlight the importance of considering the proper comparison blocks when assessing the impact of business heterogeneity on crime.
TABLE 8. Instrumental variable models for several types of crime—including interactions of business heterogeneity and residential population.
Variable Aggravated Assault Robbery Burglary Motor Vehicle Theft Larceny
Total businesses .849** .892** .703** .930** 1.415**
(16.66) (18.66) (21.90) (24.14) (37.18)
Total businesses squared −.086** −.069** −.046** −.097** −.113**
−(8.16) −(6.82) −(7.10) −(11.49) −(16.07)
Business heterogeneity 2.893** 3.163** 3.128** 2.760** 2.862**
(51.71) (52.36) (77.45) (60.43) (79.11)
White-collar businesses −.761** −1.093** −.655** −.994** −1.479**
−(9.45) −(16.77) −(14.76) −(18.96) −(23.97)
Blue-collar businesses −1.968** −2.540** −.448** −.271** −1.856**
−(18.21) −(23.20) −(5.98) −(2.64) −(22.90)
White-collar businesses squared .080** .087** .045** .111** .198**
(4.68) (7.35) (5.57) (10.87) (15.31)
Blue-collar businesses squared .454** .530** .009 −.124 .352**
(9.45) (12.55) (.20) −(1.57) (8.72)
Interactions
Business heterogeneity × population within 2.5 miles −.013** −.019** −.016** −.016** −.016**
−(16.56) −(24.28) −(26.02) −(25.80) −(27.41)
Business heterogeneity × block population .115** .517** −.241** −.066** .113**
(4.13) (16.31) −(11.99) −(2.94) (5.79)
Business heterogeneity × block population squared −.070** −.108** −.023** −.042** −.072**
−(16.87) −(21.80) −(7.58) −(12.57) −(23.26)
Control variables
Concentrated disadvantage .279** .236** .097** .155** .114**
(29.94) (18.79) (12.01) (15.31) (8.33)
Residential stability .001 −.012** .000 −.009** −.012**
(.70) −(5.60) −(.17) −(6.94) −(6.28)
Racial/ethnic heterogeneity −.067 .121* .414** .109** .212**
−(1.65) (2.53) (14.34) (3.29) (7.49)
Percent Black .020** .019** .009** .011** .002**
(52.88) (36.72) (24.46) (25.48) (2.86)
Percent Latino .009** .009** .001** .009** −.004**
(26.18) (23.34) (5.20) (22.81) −(12.02)
Percent Asian −.001* .001 .001** .002** −.003**
−(2.33) (.77) (3.50) (4.48) −(7.31)
Percent vacant units −.009** −.004** −.007** −.004** −.002**
−(11.21) −(5.22) −(12.86) −(6.57) −(2.62)
Percent aged 16–29 .001 .001 .001 .006** .004**
(1.92) (.72) (1.88) (8.01) (3.65)
Population (logged) −.232** −.416** −.045** −.301** −.393**
−(16.43) −(23.77) −(4.53) −(27.40) −(39.26)
Population squared .097** .091** .071** .103** .106**
(46.22) (32.21) (48.78) (61.87) (70.64)
Population within 2.5 miles (exponential decay) .006** .023** .002** .011** .005**
(15.35) (51.92) (5.87) (34.41) (19.54)
Tweets (logged) .037** .103** .090** .055** .215**
(10.05) (24.25) (34.81) (18.30) (92.50)
Intercept −2.823** −3.929** −2.053** −2.823** −.847**
−(49.16) −(62.81) −(45.69) −(54.45) −(11.29)
BIC 165,002 108,960 270,923 209,457 435,696
Pseudo R2 .229 .294 .192 .235 .173
Note. t values in parentheses. N = 187,714 blocks.
*P < 0.05.
**P < 0.01 (two-tailed test).
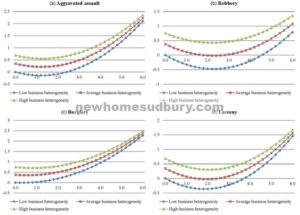
Marginal relationship between street segment logged population and crime, based on level of business heterogeneity. [Colour figure can be viewed at wileyonlinelibrary.com]
Note. N = 187,714 blocks.
A negative coefficient for the interaction between business heterogeneity and population in the surrounding area may capture potential offenders (Hipp, 2016). When plotted, it shows that the positive relationship between population in the surrounding area and crime is strongest in blocks with low business heterogeneity, whereas the relationship weakens in high heterogeneity blocks (not shown). Although this finding is consistent with Jacobs (1961), it nonetheless was the case in these figures that the positive relationship between business heterogeneity and crime dwarfs the impact of population in the surrounding environment.
In a final set of models, we tested whether concentrated disadvantage moderated the relationship between business heterogeneity and crime. Only modest evidence was found for such an effect when we included this interaction. The negative coefficient indicated that even though a positive relationship exists between business heterogeneity and crime in all neighborhoods, it is strongest in low-disadvantage neighborhoods and weaker, but still positive, in high-disadvantage neighborhoods. Nonetheless, based on the modest size of the effects, the size of the block population seems to matter more for moderating the impact of business heterogeneity than the economic resources of that population. This finding is notable given that existing research often tests for a moderating effect of concentrated disadvantage (Hipp et al., 2022; Stucky & Ottensmann, 2009; Wo, 2019).
5 DISCUSSION
This study has explored how businesses are related to where crime occurs across the micro unit of blocks in Southern California. A key implication is that the context of businesses has important consequences for understanding where crime occurs. Whereas some existing studies have focused on one type of business—such as bars—our results highlight that understanding the fuller context of businesses on a block is necessary. Failing to account for this broader context can yield biased estimates, as we described that the coefficients for bars and liquor stores were sometimes overinflated by nearly 100% in models mimicking the typical strategy in the literature. Given that businesses are always part of an environmental context, an important takeaway is the need to account for this context.
One important finding was that the information provided by specific business types for where crime occurs was limited after accounting for the general context of businesses on a block. They are not completely unimportant, however—we found that more crime does indeed occur on blocks with certain types of businesses, even after controlling for the general business context. Nonetheless, the size of this effect was small, and certainly much smaller than that found in existing research that has tested a single business type and not simultaneously accounted for the business context of the block. Future work might identify narrower categories of businesses more strongly related to crime, but theoretical guidance is needed for such a strategy. It also may be that the quality of place management at a location is what matters (Linning & Eck, 2021), implying that categories of businesses are not important. We cannot adjudicate this question here. One observation is that if certain business types are at least somewhat more important for explaining where crime occurs, then this information might be incorporated into a measure of business heterogeneity; nonetheless, we are agnostic on whether this approach would truly improve the predictive performance of a business mixing measure.
This idea leads to one of the most pronounced findings of the study, which is the strong positive relationship between business heterogeneity and crime. We have pointed out that even though Jacobs (1961) posited that such business mixing would make the environment more inviting—which it may in fact do—her conclusion that this will result in reduced crime does not necessarily follow. If greater business mixing results in more customers visiting the block, this would bring about an uncertain outcome since the increased number of guardians may not offset the increased number of potential targets and offenders. Indeed, simulation work has suggested that except at high volumes, this increased mixture would result in more crime (Birks & Davies, 2017). Our results found that this mixing was strongly related to increased crime, even when controlling for the ambient population based on geocoded tweets. We acknowledged the challenge of how to define business categories when creating a measure of business mixing—we used 31 categories of consumer-facing businesses as an initial strategy under the presumption that this would have the greatest potential to create the vibrant atmosphere Jacobs (1961) discussed. Nonetheless, categories to use in mixing measures will need to be an area of future research.
Although the strong positive relationship between business heterogeneity and crime was inconsistent with Jacobs’s (1961) theorizing, it was consistent with our discussion that business heterogeneity may affect the mixture of offenders at a location. This business mixing may increase the mixture of offenders by providing a wide variety of criminal opportunities, increasing the flow of offenders there (Song et al., 2019), or increasing the attractiveness of this as an activity node (Brantingham & Brantingham, 2016). The increase in potential offenders would increase crime incidents (Ruiter, 2017). Another possibility is that the wide variety of persons attracted to locations with many different businesses may allow offenders to blend into the environment better, which would also result in more crime. We could not test these potential mechanisms or other possible ones that might explain the relationship we observed. Nonetheless, the strong results we detected indicate that future research assessing whether more business mixing affects the mixture of offenders at a location would be fruitful.
Although the findings for business heterogeneity contradicted Jacobs (1961), one finding that was at least consistent with her hypothesizing was the moderating effect in which a larger residential population on the block effectively eliminates this positive relationship between business heterogeneity and crime. The implication is that this local population has the ability to provide some social control capability—whether it is because they are more interested in the scene and watching it, as Jacobs posited, or because they are simply in the environment serving as non-strangers willing to directly provide social control, or because their presence provides indirect social control as passive guardians. Regardless, we saw that this residential population could strongly impact how business mixing is related to crime. Also, note that this effect swamped that of how concentrated disadvantage altered the effect of business mixing on crime. In our study, the simple presence of more residents, rather than their socioeconomic status, was the important factor.
We acknowledge some limitations to this study. Although we had a large sample of blocks in a massive region, we do not know the extent to which our results will generalize to other locations. Furthermore, the fact that we had cross-sectional data only allows us to observe relationships, and we cannot say whether we observed causal effects. We did, however, use instrumental variables to address potential endogeneity for our business-related measures. Relatedly, we did not measure the potential mechanisms, so these patterns can only be consistent with expectations, but do not demonstrate why the relationships exist. Nonetheless, understanding which business environment is more likely to be associated with more crime incidents can be useful for police agencies considering where to allocate scarce resources. We used blocks as units of analysis, which, although appropriate for our setting, may not be appropriate in rural areas or cities outside of North America.
One policy implication of our findings is that police agencies may wish to carefully monitor the placement in the city of new commercial developments containing a mixture of businesses. Such locations may be at more risk of crime increases. Nonetheless, we are cautious in policy recommendations given that we are uncertain as to the mechanisms involved. It will be important to understand why locations with more business mixing experience more crime. Depending on what the actual mechanism is—whether a gathering spot for potential offenders, a mixture of offenders being attracted, and so on—will likely guide the actual policy implications.
In conclusion, we highlight the need for scholars to consider the context in which businesses exist on local blocks. Whereas studies have often focused on the relationship between a certain type of business and crime, our results highlight that we need to account for the context of the other businesses located on the same block. We found that measuring the number of businesses in broad categories provides considerable information about the location of crime, and measuring specific business types provides little additional information. A particularly important result was that the level of mixing of businesses on a block has a strong positive relationship with the amount of crime on the block—this feature of the environment is under-considered and highlights that the context of the environment matters more than any specific businesses. Finally, the residential population of the block moderated this relationship of business mixing, further highlighting that researchers need to give more theoretical consideration to how to measure the context in which these businesses occur.
APPENDIX
Here we provide the equations for the theoretical section of this article. These equations consider a stylized scenario with many types of businesses (we will assume 100) and how they might be related to crime in the local area. For the moment, we will ignore how these relationships might differ over various crime types. In the models described in this appendix, the outcome variable (y) will be crime incidents (of an unspecified type). The total number of businesses (XN) is a summation of all 100 types of businesses. The count of each business type can be expressed as X1, X2 … X100.
To assume a linear positive relationship between businesses and crime implies the following model:13
(1)
where the number of crime incidents is a function of the total number of businesses in the local environment. Note that an assumption of this model is that all businesses equally impact the number of crime incidents, and therefore, each additional business (of any type) will increase crime incidents β units (assuming a positive coefficient).
Researchers who focus on one type of business establishment specify the following model:
(2)
where each additional business of X1 (e.g., bars) will increase crime incidents β units. Note that an assumption of this model is that other businesses have zero impact on crime.
A strategy that is not used would specify a model in which variables are included for the count of each of the 100 types of businesses:
(3)
This model relaxes the assumption of model 1 that all business types have the same impact on crime, and now each business type is allowed to have its own coefficient for its impact on crime (β1, β2, etc.). A downside to this model is that it estimates many coefficients—and even more, if one categorizes businesses even more finely. If one could reduce the number of business types to fewer categories, one could more easily estimate this model. Note that it is preferred that the business types aggregated into each broader category would have similar relationships with crime—that is, similar coefficients from model 3 since the aggregated model would estimate a single coefficient for each aggregation of business types.14 Nonetheless, much more theoretical guidance would be necessary for such categorization.
Another model that would focus on a particular business type (X1), but account for all other business types having the same impact, would imply the following model:
(4)
where β1 captures the effect of the business type of interest and β2 captures the effect of all other business types (which are all assumed to have the same effect on crime). In this model, XN would be a count of all business types except for X1.15 This model is an improvement over Equation (2), as we do not have to assume that β2 is zero, which Equation (2) assumes. We are assuming, however, that all other businesses have the same relationship with crime, that is, β2.
One classification of businesses is to distinguish between consumer-facing and non–consumer-facing businesses. Consumer-facing businesses by definition draw customers and, therefore, typically provide more crime opportunities. Nonconsumer businesses (such as office buildings for white-collar workers or factories for blue-collar workers) do not attract customers and, therefore, provide fewer crime opportunities. Therefore, the prior model is modified as follows:
(5)
where XNCF are non–consumer-facing businesses and XCF are consumer-facing businesses, which have distinct coefficients capturing their distinct relationships with crime.
Is the relationship between businesses and crime nonlinear?
If a nonlinear relationship exists between businesses and crime, we could specify the following model:
(6)
where X2N is the square of the number of total businesses, and the β2 coefficient would presumably be negative, showing that crime begins to fall when many businesses are located in an area (due to the presence of more guardians).
Equation (6) assumes that all business types equally impact crime, which could be relaxed by distinguishing between consumer-facing and non–consumer-facing businesses, yielding the following model:
(7)
where XN includes both consumer- and non–consumer-facing businesses and X2N is the squared version of this measure, whereas XNCF is the number of non–consumer-facing businesses and X2NCF is the squared version of this measure. This parameterization allows capturing the nonlinear effect of the total number of businesses, and β3 and β4 capture the difference between consumer- and non–consumer-facing businesses for this nonlinear effect. Interpreting the relationship between non–consumer-facing businesses and crime is more complicated as it includes summing all four β parameters, but it is trivial. This parameterization appropriately captures the nonlinear effect of all businesses (which would not be the case if we instead included a measure of consumer-facing businesses and its quadratic rather than total businesses).
To account for one particular business type (X1) along with the nonlinearity of all businesses implies specifying the following model:
(8)
where Equation (4) has been extended by accounting for this nonlinear effect of total businesses, XN is all businesses including X1, and X2N is the squared version of this measure. Thus, β1 captures the effect of our business type of interest on crime, accounting for the possible nonlinear impact of total businesses in the environment on crime. Note that this model assumes that the effect of our business type of interest is constant regardless of the context of total businesses (as there is just a single β1 coefficient) independent of its role in the nonlinearity effect of all businesses. We could relax that assumption by including interactions of our measure with the total business environment:
(9)
where β4 and β5 capture the effects of X1 based on the total number of businesses in the environment.
As an aside, a question exists of whether XN in Equations (8) and (9) should be a count of all businesses, or of whether it should exclude X1 businesses. In this case, where we posit a nonlinear relationship between the total number of businesses and crime, excluding X1 businesses from XN will instead assume that each additional X1 business has a linear effect on crime, and that it does not contribute to the nonlinear effect of the total number of businesses. This outcome is likely implausible, and therefore, we would more likely specify XN as the total number of businesses. The implication is that β1 does not entirely capture the effect of each X1 business on crime in an environment as the business will also impact crime nonlinearly through β2 and β3 in Equation (8), as well as β4 and β5 in Equation (9). The analyst would simply need to keep this in mind when interpreting the results; nonetheless, it is likely a more theoretically justifiable model specification.
We could account for more than just one specific business type—for example, five—and could specify the following model:
(10)
where β1 through β5 capture the effects of these five business types on crime while accounting for the nonlinear effects of the total number of businesses in the environment. One could also include interactions between these five measures and the total number of businesses (and the quadratic version).
Does the relationship between a business type and crime depend on the context?
If we construct a measure of the mixture of businesses in an environment based on an Entropy measure or a Herfindahl measure (a sum of squares of proportions of different business types; XHET), a possible model would be as follows:
(11)
where β1 captures the effect of our specific business type of interest (X1), β2 captures the effect of the total number of businesses, β3 captures the effect of the mixture of businesses, and β4 captures the effect of the interaction between the number of businesses and this mixture. This interaction term captures the possibility that this mixing may impact crime differently when more businesses are located in the environment, as described earlier. If one felt nonlinear effects of the total number of businesses also occurred—in addition to this mixing effect—one could include a quadratic version of XN and its interaction with XHET.
A limitation of the XHET measure is that it assumes that any mixing of businesses is the same as any other business mixing. If one had theoretical reasons to expect that certain mixtures of businesses have stronger impacts on crime than others, one might wish to measure those directly.
Biographies
John R. Hipp is a professor in the Department of Criminology, Law and Society and the Department of Sociology at the University of California–Irvine. His research interests focus on how neighborhoods change over time, how that change both affects and is affected by neighborhood crime, and the role networks and institutions play in that change.
Cheyenne Hodgen is a PhD student in the Department of Criminology, Law and Society at the University of California–Irvine. Her research interests include spatiotemporal patterns of crime and the relationship between the built environment and crime.
Leave a Reply